Table of Contents
Machine Learning Safety
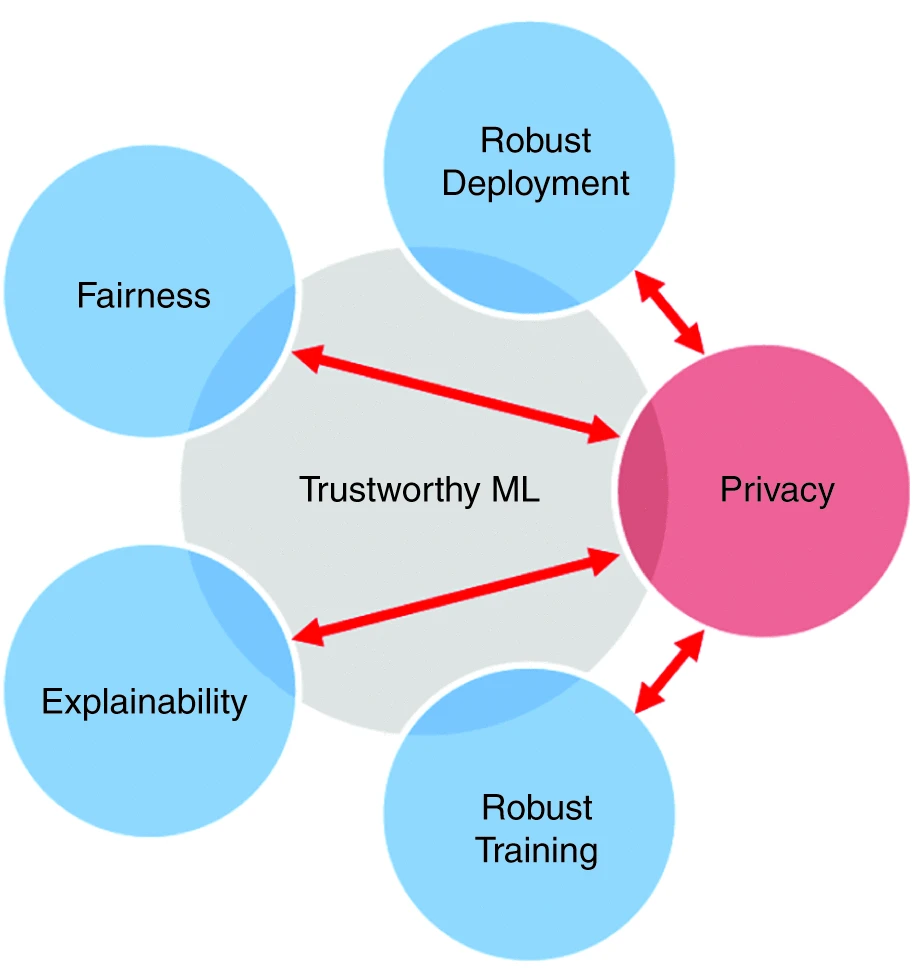
While machine learning (ML) has shown remarkable success in many applications such as content recommendation on social media platforms, medical image diagnosis, and autonomous driving, there is a growing concern regarding the potential safety hazards coming with ML. As exemplified in Figure 1, people are interested in multi-facets evaulation of machine learning models besides accuracy.
My current research focuses on three main aspects: data privacy, model privacy, and model fairness.
Data and Model Privacy
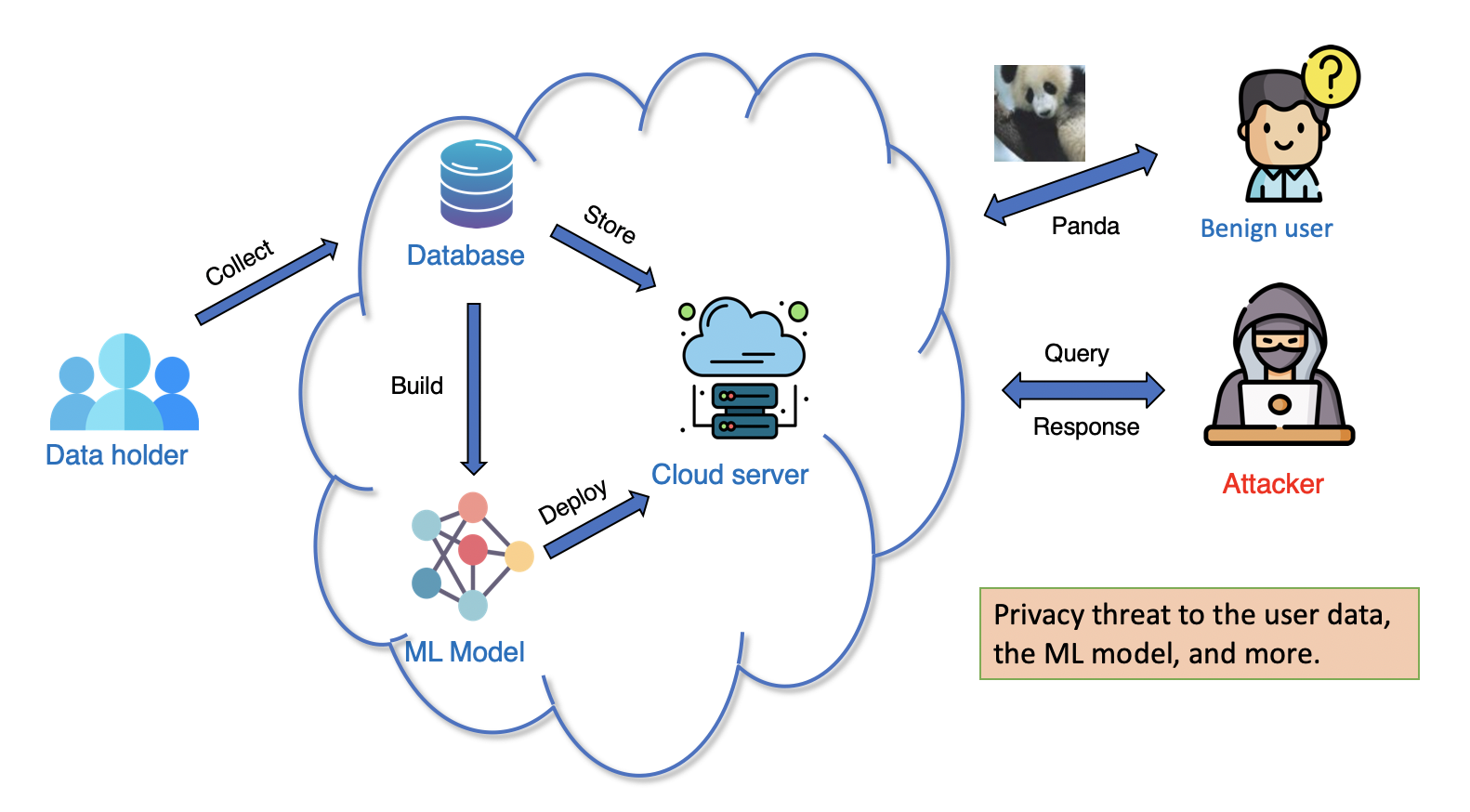
The privacy threat is inconspicuous but widely exists in our daily lives. We risk privacy leakage for every step in the machine learning life cycle, such as collection, storage, release, and analysis, as illustrated in Figure 2. Our goal is to develop privacy-preserving methods with built-in privacy guarantees, especially for the collected data and built models.
Data privacy pertains to the protection of sensitive information collected to build ML models. We have proposed a novel approach called Subset Privacy
for protecting categorical data and developed an open-sourced software implementation [4, 8]. We have demonstrated its usage in multiple learning tasks and shown its potential to be useful in a wide range of fields beyond statistics due to its unique user-friendly implementation.
In addition to data privacy, the reliability and security of the ML models built from the collected data are of paramount concern, which we refer to as model privacy. We have formulated the Model Privacy
framework that can be applied to analyze multiple model attacks including stealing and backdoor attacks [3, 7, 9, 10].
Model Fairness
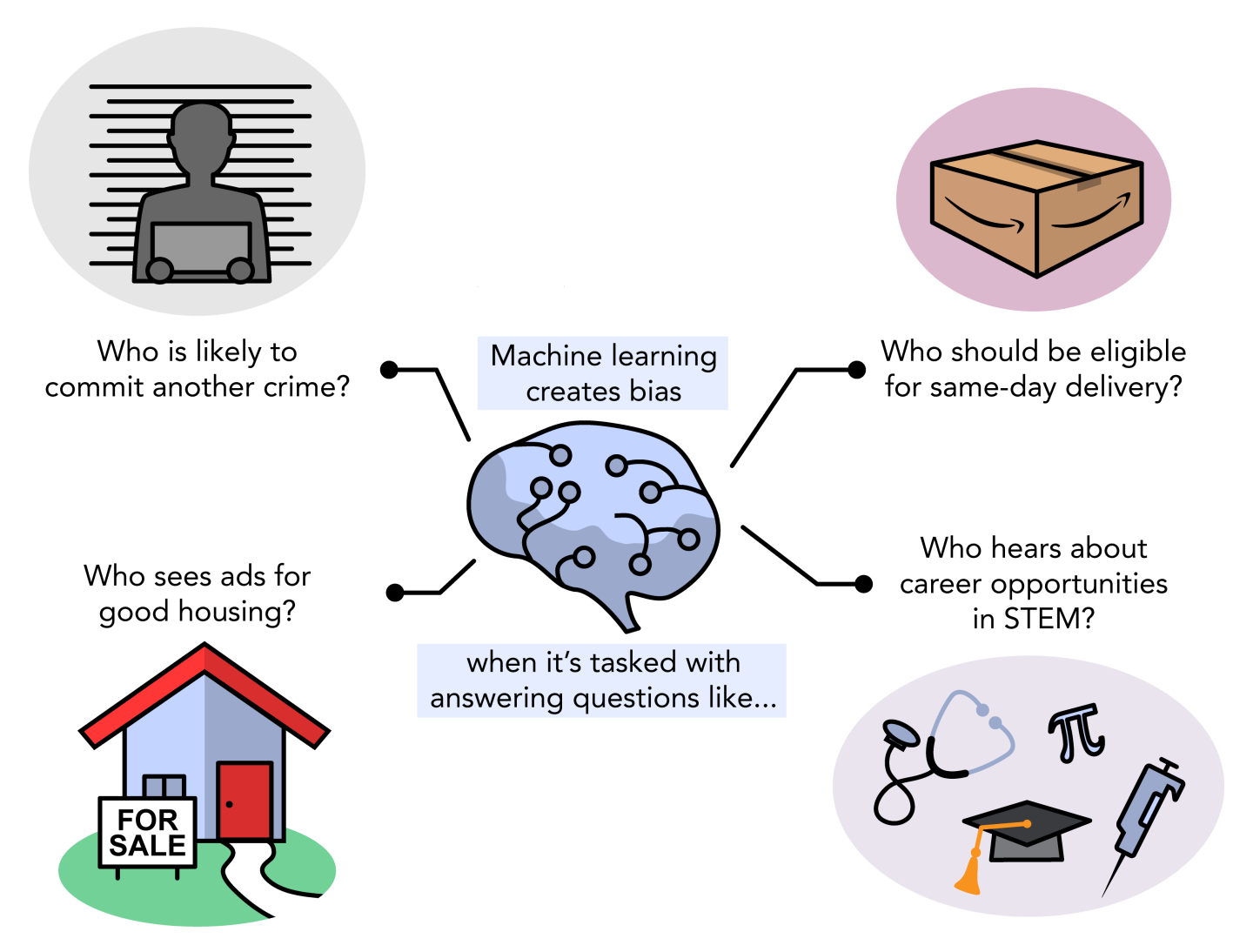
The fairness of the ML model has attracted significant attention nowadays, especially in areas such as criminal justice and banking. It is well-known that ML models may inadvertently be unfair. For example, the COMPAS algorithm, which assigns recidivism risk scores to defendants based on their criminal history and demographic attributes, was found to have a significantly higher false positive rate for black defendants than white defendants, thereby violating the principle of equity on the basis of race.
Our goal is to build a model that makes equitable decisions for different groups in the population. We have identified the conditions under which a broad class of distributed ML algorithms can produce fair models. Additionally, we have proposed a new algorithm that directly optimizes model fairness with theoretical guarantees [5].
Other Safety Aspects
Explainability: We studied the effect of the LASSO regularization on variable selection in terms of neural networks [2]. Our understanding indicates that neural networks can consistently select significant variable while achieving satisfactory accuracy.
Model compression: An emerging demand of machine learning models is to reduce the model size while retaining a comparable prediction performance to address limitations in computation and memory. We investigated a fundamental problem in model pruning: quantifying how much one can prune a model with theoretically guaranteed accuracy degradation. Insipred by the developed theory, we proposed a data-driven, adaptive pruning algorithm [1, 6].
Publications
Peer-reviewed
* indicates equal contributions, † indicates corresponding author(s)
-
Ganghua Wang†, Ali Payani, Myungjin Lee, and Ramana Kompella. “Federated learning with group bias mitigation: beyond local fairness”. TMLR (2024) [pdf]
-
Ganghua Wang*, Xun Xian*, Jayanth Srinivasa, Ashish Kundu, Xuan Bi, Mingyi Hong, Yuhong Yang, and Jie Ding†. “Demystifying Poisoning Backdoor Attacks from a Statistical Perspective”. ICLR (2024). [pdf]
-
Xun Xian, Ganghua Wang, Jayanth Srinivasa, Ashish Kundu, Xuan Bi, Mingyi Hong, and Jie Ding†. “A Unified Framework for Inference-Stage Backdoor Defenses”. Proc. NeurIPS (2023) [pdf]
-
Enmao Diao*, Ganghua Wang*, Jie Ding, Yuhong Yang, and Vahid Tarokh†. “Pruning deep neural networks from a sparsity perspective”. Proc. ICLR (2023) [pdf]
-
Gen Li, Ganghua Wang, and Jie Ding†. “Provable Identifiability of ReLU Neural Networks via LASSO Regularization”. IEEE Trans. Inf. Theory (2023) [pdf]
-
Xun Xian*, Ganghua Wang*, Jayanth Srinivasa, Ashish Kundu, Xuan Bi, Mingyi Hong, and Jie Ding†. “Understanding backdoor attacks through the adaptability hypothesis”. Proc. ICML (2023). [pdf]
-
Ganghua Wang, Jie Ding, and Yuhong Yang†. “Regression with Set-Valued Categorical Predictors”. Statistica Sinica, (2022) [pdf]
Under Review
-
Xun Xian, Ganghua Wang, Xuan Bi, Jayanth Srinivasa, Ashish Kundu, Charles Fleming, Mingyi Hong, and Jie Ding†. “On the Vulnerability of Applying Retrieval-Augmented Generation within Knowledge-Intensive Application Domains” Proc. ICLR (2025)
-
Xun Xian, Ganghua Wang, Xuan Bi, Jayanth Srinivasa, Ashish Kundu, Mingyi Hong, and Jie Ding†. “RAW: A Robust and Agile Plug-and-Play Watermark Framework for AI-Generated Images with Provable Guarantees” Proc. ICLR (2025)
-
Wenjing Yang*, Ganghua Wang*, Jie Ding, and Yuhong Yang†. “A Theoretical Understanding of Neural Network Compression from Sparse Linear Approximation”. arXiv preprint (2024) [pdf]
-
Ganghua Wang and Jie Ding†. “Subset Privacy: Draw from an Obfuscated Urn”. arXiv preprint (2024). [pdf]
Manuscript
[10] Ganghua Wang, Yuhong Yang, and Jie Ding†. “Model Privacy: A Framework to Understand Model Stealing Attack and Defense”. Manuscript